Enter the 15% Club: how to make sure your AI project does not fail
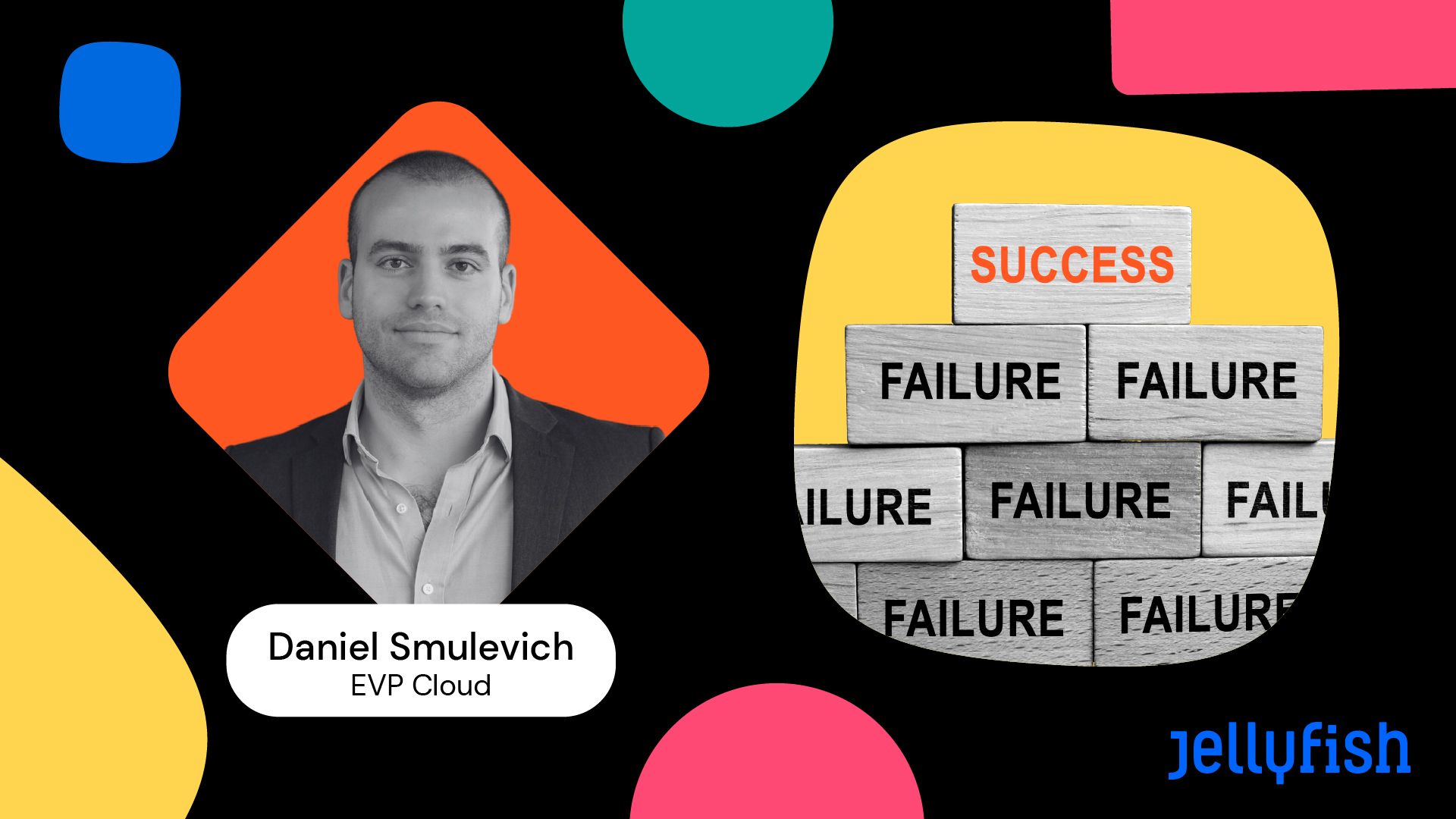
According to Gartner,
85% of AI projects fail. Daniel Smulevich, EVP Cloud at Jellyfish, explains why the failure rate is so high, and how to avoid becoming one of the 85%
Given the hype around AI, it’s not surprising that everyone wants a piece of the action. With its promise of automating manual tasks, processing unfathomable amounts of data in seconds, and freeing up people to spend time doing the things that use their unique talents, AI is high on the agenda for virtually all businesses with the resources to invest in it.
When it comes to investing in AI, companies can take three paths. The first is to buy a “pre-canned solution,” where the model is already built. The business just needs to input the data and then process the output. This is the simplest, lowest-risk, but also least-customisable way of deploying AI in a business.
At the other end of the spectrum is where the company builds something bespoke, using existing machine learning frameworks and infrastructure, but building their own model on top of that. This offers maximum customisation, but also requires the highest levels of expertise and investment, so tends to be the route taken only by the largest enterprises.
In between these two extremes, there’s a middle ground, where the business takes the existing libraries and frameworks and tweaks them to suit their own use cases, offering a useful degree of customisation, without the levels of investment and expertise required to build a fully bespoke solution.
This is the most common scenario, and it’s also where most companies fail. In fact, in an article in the Harvard Business Review late last year, Iavor Bojinov, Assistant Professor at Harvard Business School, noted that some estimates
place the failure rate of AI projects as high as 80%. That’s only a 5% improvement on 2018, when Gartner reported a failure rate of 85%. So why is this figure still so high, and what can be done to prevent failure and ensure success?
Business case
The first reason, in my experience, is that very few projects have a solid business case. No one made the effort to define what success would look like, and where projects are evaluated; there are inflated expectations because the KPIs are too fluffy; and no one has considered what the drivers of the KPI should be. If the KPI is to increase profits, will this be achieved by converting more customers, increasing the average order value, reducing the number of returns, or all of these, combined with many other potential contributing factors? And for each of these, what actions will drive that outcome?
Often, there is also too much emphasis on the cost, and not enough on the likely returns. There are three elements to consider here: cost, risk and return. The risk is the probability of the return not being realised, but it’s often not considered. If you don’t document the likely returns - and how the risk of not achieving them might be reduced by spending a little more - the only line item that ever comes under scrutiny is the cost. The outcome of this isolated focus on the cost is often a budget cut.
The second problem is that marketers need to speak the language of IT, and vice versa, but because IT has become such a key component of every business department, the balance of power has shifted towards IT. So I’d suggest marketers need to lean in and get more involved with IT - learn to speak their language, and help them to understand yours.
It’s very rare that we see marketing teams with IT people working within them - even though no marketing AI project will deliver meaningful value without help from IT. But too often, involvement tends to be on a project-by-project basis, and things get stuck for months because IT has competing priorities elsewhere.
This is exacerbated by the fact that the half-life of tech projects has reduced considerably. Years ago, companies were happy to invest in IT projects, safe in the knowledge that they would give years of value. Now, that payback window is much shorter. If it takes a year to build something, realistically you need to have started planning the next iteration even before it’s finished.
This ties in to the maintenance issue, and what happens after you have built the solution. Building these systems is not a once-and-done thing - companies need to put plans in place to keep them up to date. Similarly, whenever a new system is developed, it’s vital that people are trained to use it - and taught why they should bother to do so. Otherwise, it won’t get widespread adoption, and the investment is wasted.
Solid foundations
Now, you could argue that all the above applies to any IT project, and in a sense that’s true. But it’s especially important when it comes to building and deploying new AI projects. With the latest wave of generative AI development in particular, there is still only limited evidence of what works and what doesn’t - there’s a lot of talk, but relatively few case studies.
So it’s even more important to get the basics right and start from solid foundations, with a clear plan and vision for what the project is going to cost, what it’s going to deliver, and across what time frame.
The final point to consider is that
any AI system is only as good as the data it’s fed with. In my experience, most companies who deploy AI systems do not feed it with the most valuable data - the data that is unique to their business. That might be location data around where their customers live, what type of car they drive, and their household income; or business data, like which products make the best margin; or category data, specific and vital to the vertical they operate in - such as, for a travel company, how long in advance of a holiday do people start doing their research?
At Jellyfish, we have developed what we call the MMI Blueprint—Mix, Match, Integrate—to help companies identify this data and use it in their AI systems. The Blueprint also helps establish the KPIs that are so important in defining what the system is intended to deliver.
The MMI Blueprint provides a solid foundation for deploying an AI system, and then ensures it is fed with the right sort of data once it’s up and running. All of which means it stands a much greater chance of being one of the 15%, rather than just another among the 85%.
Also published in:
Martech Outlook